Statistical Analysis of Wasserstein GANs with Applications to Time Series Forecasting
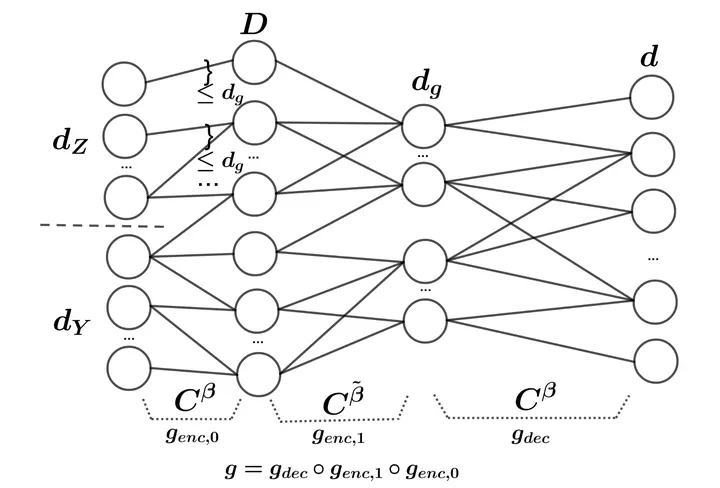
Publication
arXiv:2011.03074
We analyse Wasserstein GANs statistically. Interestingly, our excess risk bound for unconditional WGANS (from 2020!) captures a key advantage of generative models: Since we can generate as many samples as we want, the generalization error is only limited by the critic network and the dataset size. If we generate enough samples (and assume to find a global optimizer), the generator network may be arbitrarily large. We also show that large critic networks metrize weak convergence, that is they are able to distinguish arbitrary pairs of distributions and guide the generator to reproduce the data distribution.